Vehicle Telematics Data Analysis
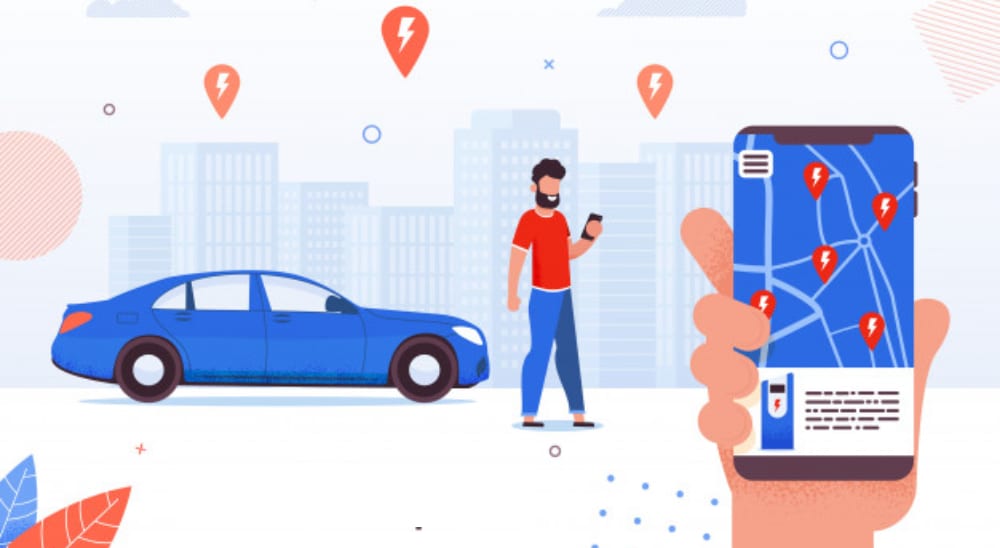
Background
Telematics in the automotive sector is a quantitative method of monitoring a moving asset (usually a car or truck) with data from onboard GPS and sensor-based diagnostics system -- sometimes combined with information from other sources like weather forecast, road or traffic report. Telematics data analysis is used by a wide range of actors from insurers to regulators to manufacturers to reduce costs, improve efficiencies, comply regulations and set up new services like driving behavior-based insurance, car concierge systems and fleet management
Examples
The focus of telematics analysis could vary, but one of the most general addresses the driving behavior of the vehicle users. Individual driver habits evidently and prominently affect fleet asset condition and fuel economy, safety, ride comfort, environmental impact, road congestion among other things. Telematics is also able to measure unwelcomed events like in-vehicle consumption of entertainment content, excessive speed, unauthorized or unsafe stopping, sudden lane changes, speed limit violation. We concentrate here to complex data driven applications for corporate vehicle users and fleet managers in performance optimization and alerts for undesirable events.
User categorization
Considering the person of the driver, who is a decisive factor in the driving process, we could apply classification from various aspects.

One such aspect is driving behavior: studying the factors associated with driving styles could help fleet managers to understand and measure drivers’ effect of their cars with all implications to the physical conditions, fuel, and insurance costs related to the consequences of specific driver’s specific driving habits.
In the analysis we could set up categories like “safe driver”, “aggressive driver”, “good fuel economy driver”, “road friendly driver” etc. or we can recognize to specific dangerous events like inattentiveness, drunkenness. Driving styles could vary a lot depending on the country’s cultural, infrastructural and meteorological conditions, but AI is still able to draw general conclusions for specific enquiries.
Having these insights stakeholders could encourage positive, discourage negative behaviors accordingly or score their best/worst drivers. An interesting extension here are game theory and game approach that can be used for driver coaching by rewarding appropriate driving habits, organizing personalized driver training, long term involvement of drivers into expected behavior is being desirable.
Another useful method is driver recognition (or identification): similar classification task can be applied for another purpose with the goal of security. Due to the definitive and measurable nature of the driving process, analyzing driving behavior is a way of collecting a kind of indirect biometrics data. As such, after learning characteristics from verified historical data can be compared with analysis of real time data and in case of anomaly — ie. the actual driving style differs from the persons who is supposed to drive the car — real time alert could be sent to the management.
Added values (Why AI/ML/DL): tracking and giving feedback on driver habits in order to extend vehicles’ lifespan and prevent accidents and other kind of related damages.
Proposed tech stack: Linux, Python (Anaconda), Scikit-learn, TensorFlow, PyTorch,
Search
Tech Stack
Android
Angular
Apache
Artificial Intelligence
Business Analysis
C++
Centura
Cloud
Docker
EAPware
Firebase
Gitlab
Industry 4.0
IoT
Java
JavaScript
JIRA
Linux
Load Test
M2M
MariaDB
Microservice
NLTK
Node.js
OpenWRT
Oracle
PHP
PLC programming
Python
PyTorch
RASA
Scikit-learn
TensorFlow
Test Automation